Evaluating the Robustness and Efficiency of Estimators for Informative Covariate Censoring
Tanya Garcia
Associate Professor, University of North Carolina-Chapel Hill
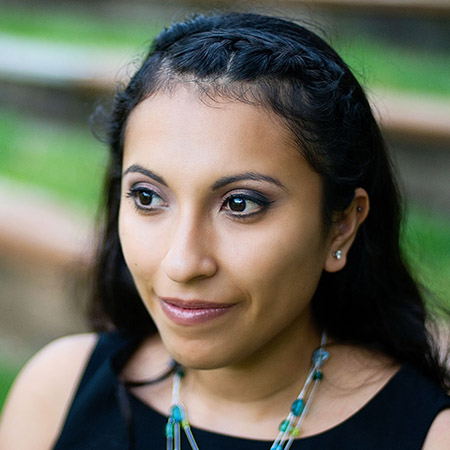
Abstract: While right-censored time-to-event outcomes have been studied for decades, handling time-to-event covariates, also known as censored covariates, is now of growing interest. So far, the literature has assumed that the covariate censoring is noninformative, i.e., event time is independent of censoring time, and has yet to establish methods when covariate censoring is in fact informative. Here, we develop different methods capable of handling both informative and noninformative covariate censoring, namely a complete case, inverse probability weighting, maximum likelihood, and augmented weighting estimators. We establish the robustness and efficiency properties of these estimators in terms of when these estimators remain consistent despite incorrect distributional assumptions. We further derive their asymptotic properties and provide a hypothesis test that can be used in practice to check if the covariate censoring is truly informative or noninformative. Empirical studies demonstrate the robustness properties of each method. All methods are applied to Huntington disease observational study data to analyze cognitive impairment as a function of time to clinical diagnosis.