Statistical Network Analysis: Estimation and Inference
Ji Zhu
Professor, University of Michigan
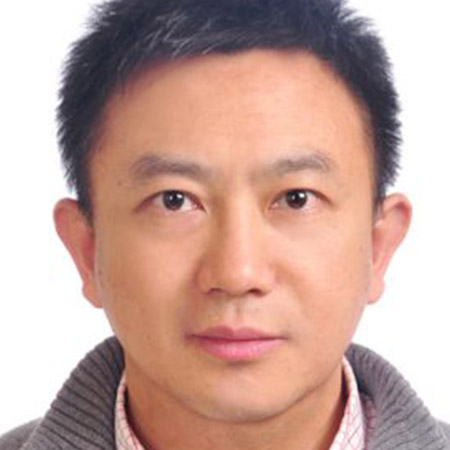
Abstract: Recent advances in computing and measurement technologies have led to an explosion in the amount of data with network structures in a variety of fields including social networks, biological networks, transportation networks, the World Wide Web, and so on. This creates a compelling need to understand the generative mechanism of these networks and to explore various characteristics of the network structures in a principled way. Latent space models are powerful statistical tools for modeling and understanding network data. While the importance of accounting for uncertainty in network analysis is well recognized, current literature predominantly focuses on point estimation and prediction, leaving the statistical inference of latent space network models an open question. In this talk, I will present some of our recent work that aims to fill this gap by providing a general framework for analyzing the theoretical properties of the maximum likelihood estimators for latent space network models. In particular, we establish uniform consistency and individual asymptotic distribution results for latent space network models with a broad range of link functions and edge types. Furthermore, the proposed framework enables us to generalize our results to the sparse and dependent-edge scenarios. Our theories are supported by simulation studies and have the potential to be applied in downstream inferences, such as link prediction and network-assisted supervised learning.